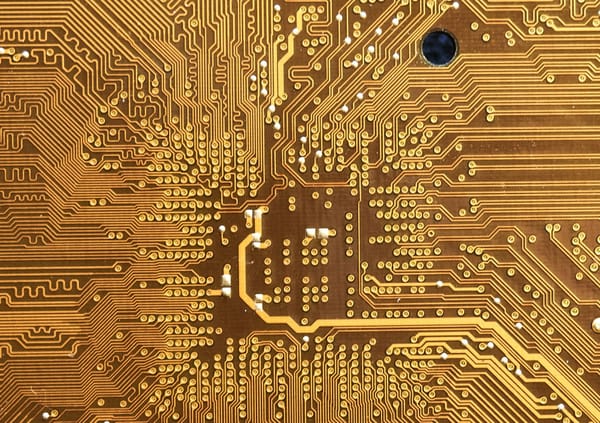
artificial intelligence
Who funds quantum research?
An odd little detail in a Physics World piece on Microsoft’s claim to have made a working topological qubit: Regardless of the debate about the results and how they have been announced, researchers are supportive of the efforts at Microsoft to produce a topological quantum computer. “As a scientist