Learning with ChatGPT
I have access to the premium version of ChatGPT, and every day I ask it a few questions about concepts in physics that I’d like to know more about. Yesterday, for example, I learnt the difference between quenching and annealing…
Is there an opposite phenomenon for quenching?
Yes! The opposite of quenching would be processes that allow gradual relaxation, equilibration, or annealing rather than rapid freezing. Some terms that describe this include:
Annealing (Thermal and Simulated) — In metallurgy and physics, annealing is the process of slow cooling a material so it can reach a more ordered, lower-energy state.
In simulated annealing (used in optimization and machine learning), a system is gradually "cooled" to allow it to find a global minimum rather than getting trapped in local minima (which can happen in quenching).
Slow Equilibration / Adiabatic Processes — Unlike quenching, which often leads to metastable states, slow thermalization allows the system to reach true equilibrium.
Adiabatic processes in quantum mechanics and condensed matter physics allow the system to evolve slowly so that it remains in a well-defined state.
Supercooling vs. Slow Nucleation — When liquids are rapidly cooled (quenched), they might bypass crystallization and form a glass. In contrast, if cooling is slow, proper nucleation occurs, leading to a well-ordered crystal.
Self-Organization & Aging in Complex Systems — Some complex systems exhibit aging (e.g., glasses, biological systems) where they slowly evolve toward an ordered or more stable configuration over time, in contrast to being frozen in a quenched state.
… and later about the Weierstrass function.
Please explain the Weierstrass function to me.
The Weierstrass function is a famous example of a continuous but nowhere differentiable function. It is defined as:
[ W(x) = \sum_{n=0}{\infty} an \cos(bn \pi x) ]
where:
- ( 0 < a < 1 ) (controls decay)
- ( b ) is an odd integer ( > 1 ) (controls frequency)
- The product ( ab > 1 + \frac{3\pi}{2} ) ensures nowhere differentiability.
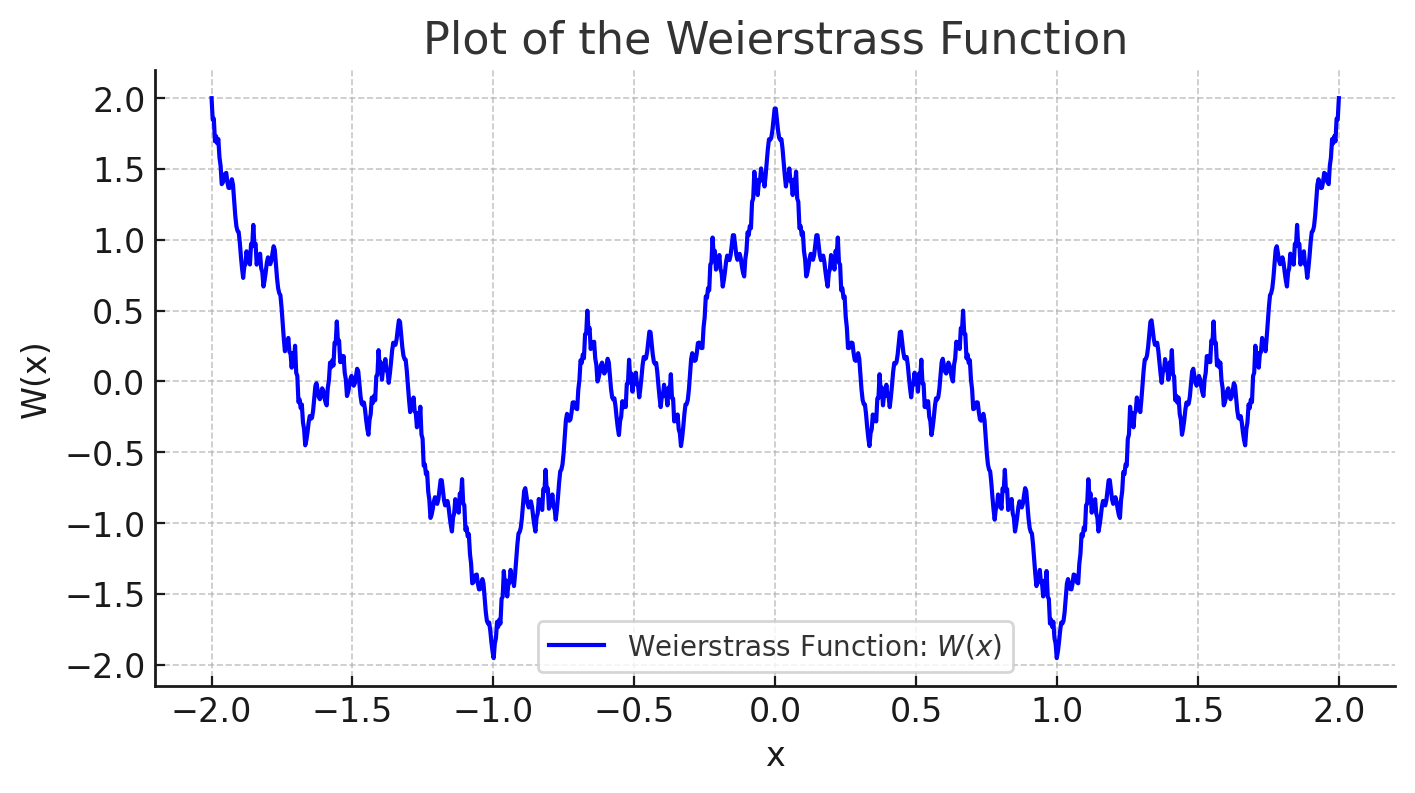
Key Properties
Continuous Everywhere — Despite its wild oscillations, ( W(x) ) is continuous for all ( x ).
Nowhere Differentiable — No well-defined slope exists at any point, making it an early example of a function that is continuous yet "rough" at all scales.
Self-Similarity (Fractal-like Behavior) — Since it is constructed as an infinite sum of cosine waves with exponentially increasing frequencies, it exhibits self-similarity: small-scale structures resemble larger-scale patterns.
Role in Mathematics — This function was introduced by Karl Weierstrass in the 19th century as a counterexample to the assumption that "continuous functions are differentiable almost everywhere."
I have found using ChatGPT in this way to be a valuable learning tool, and I imagine there are already people out there who are repackaging instances of ChatGPT to be autonomous ‘teachers’ for students looking to learn something.
One of my jobs at The Hindu is to get explanatory articles for concepts in (pure) science. I recently attempted one on amplifiers, which required me to explain the working of a NPN bipolar junction transistor, a device I’d until then been happy to leave behind in my high school classroom. I turned to ChatGPT, asking for a visualisation of the transistor, and it obliged. I’m a visual learner and having access to the tool made a big difference.
How does an NPN bipolar junction transistor work? Please explain with a visualisation.
An NPN bipolar junction transistor (BJT) is a type of transistor that consists of three layers of semiconductor material: two n-type layers (emitter and collector) separated by a p-type layer (base). It is commonly used for switching and amplification.
How an NPN Transistor Works
Structure & Terminals:
Emitter (E): Heavily doped n-type region that emits electrons.
Base (B): Thin, lightly doped p-type region.
Collector (C): Moderately doped n-type region that collects electrons.
Operation Principles:
When a small current is applied to the base-emitter junction (forward-biased), it allows a larger current to flow from the emitter to the collector.
The base-emitter junction acts as a diode, allowing electrons to flow into the base.
Most electrons injected from the emitter into the base are swept into the collector due to the voltage difference.
Visualization
Collector (C)
|
|
|----|----|
| N | <-- Collector (N-type)
|----|----|
|
|----|----|
| P | <-- Base (P-type, thin and lightly doped)
|----|----|
|
|----|----|
| N | <-- Emitter (N-type, heavily doped)
|----|----|
|
|
Emitter (E)
Current Flow:
Electrons flow from Emitter (E) to Collector (C).
A small Base (B) current controls a much larger Collector (C) current.
Voltage Conditions:
V_BE (Base-Emitter voltage) > 0.7V (Forward biased)
V_CE (Collector-Emitter voltage) > V_BE (Reverse biased)
Electron Flow:
→ Represents electron flow.
I_B (Base Current) is small but controls I_C (Collector Current), which is large.
( +V_C ) | | [C] ← Electrons | | | [B] → Small Base Current (I_B) | | | [E] → Large Electron Flow (I_E) | ( GND )
Working Mechanism:
The Base-Emitter junction is forward-biased, allowing electrons to move from Emitter to Base.
Most electrons are swept into the Collector due to the reverse-biased Collector-Base junction.
A small Base current (I_B) controls a large Collector current (I_C), amplifying the input signal.
I have a background in engineering plus more than a decade’s experience in spotting yellow/red flags in scientific papers, and I imagine anyone with these skills will have an easier time navigating ChatGPT’s answers. For anyone else that lacks these skills, all we need is a guarantee from OpenAI that the tool doesn’t hallucinate or that it hallucinates in specific contexts, and definitely not above a certain rate.